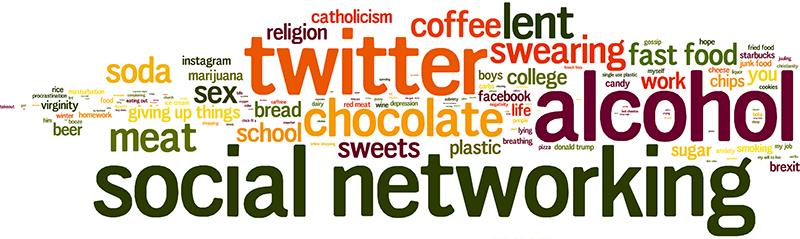
This year social networking topped the list, as it did last year, followed by alcohol, Twitter, chocolate, and, ironically, Lent. Swearing fell to #7 this year from #5 last year. With the absence of a major political or social event, 2019 was a fairly typical year for what people said they would give up for Lent.
This year, 44,291 tweets (excluding retweets) specifically mentioned giving up something, up from last year’s 29,609. In all, this year’s analysis covers 491,069 tweets, up from 427,810 last year.
Plastic
Giving up plastic has become increasingly popular in the past two years. In all, 464 tweets this year mentioned plastic of some sort, which would almost bring it into the top ten.
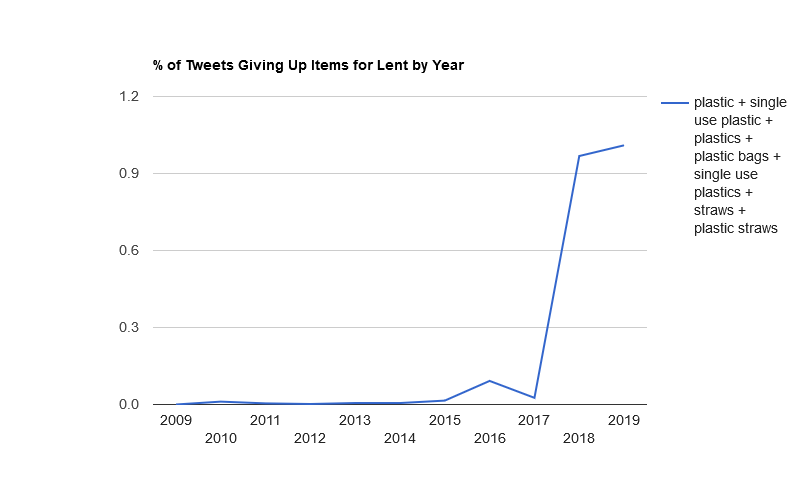
Brexit
The one major political event occurring over Ash Wednesday involved the ongoing Brexit debate. When British Prime Minister Theresa May accepted a suggestion that British lawmakers give up the EU for Lent, it led others to tweet the opposite:
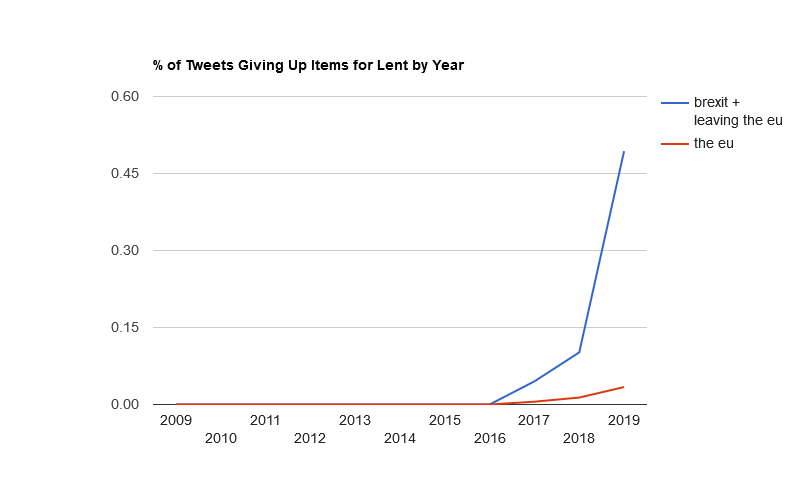
Depression and Anxiety
It was a banner year for those who said they were giving up both:
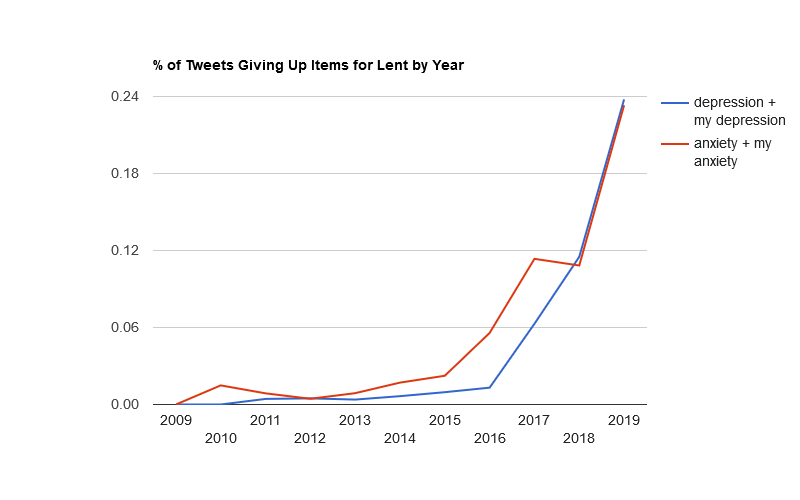
Winter
Tweets about cold weather were up this year, as they are cyclically depending on the severity of winter weather:
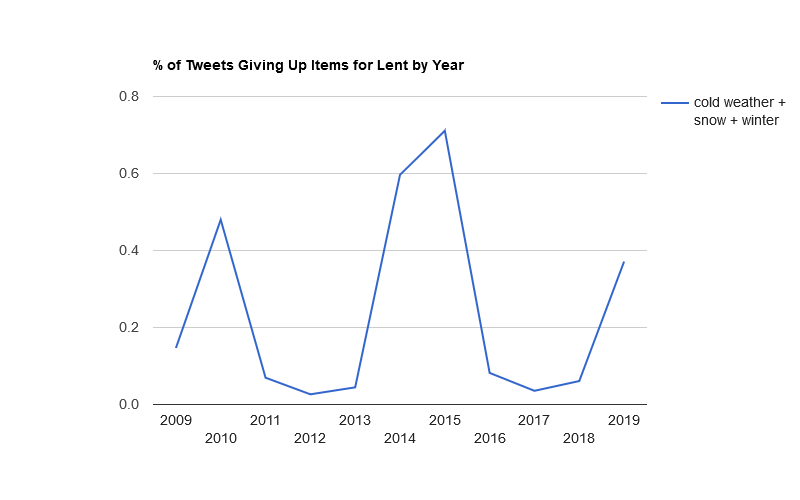
Gossip
Pope Francis this year suggested giving up gossip for Lent, leading to an increase in the number of tweets about it:
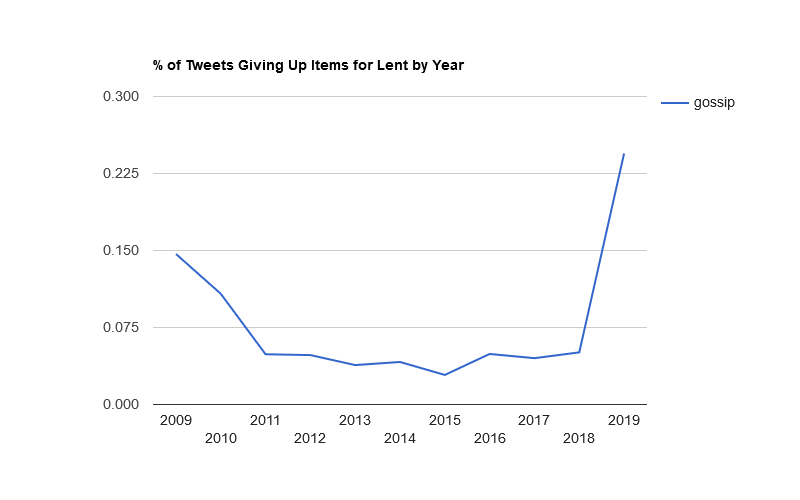
Relationships
Even though last year Ash Wednesday fell on Valentine’s Day, this year the percentage of people saying they were going to give up a significant other rose:
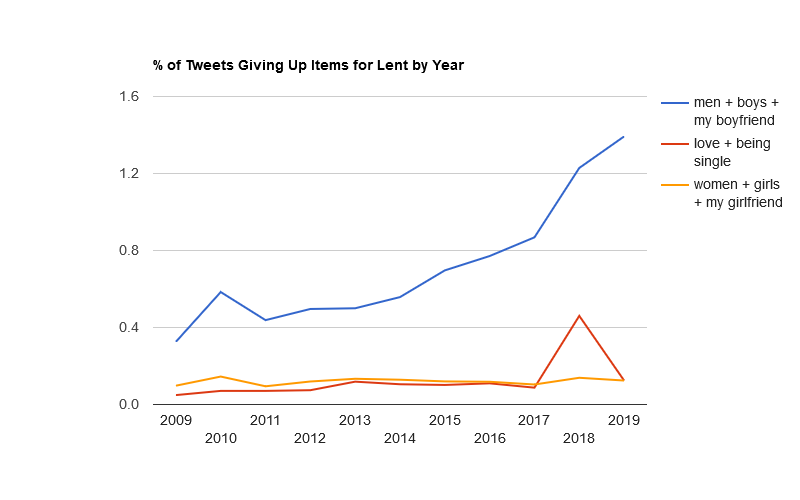
Fast Food
Chick-fil-A finally surpassed McDonald’s this year, and Chipotle continues its decline:
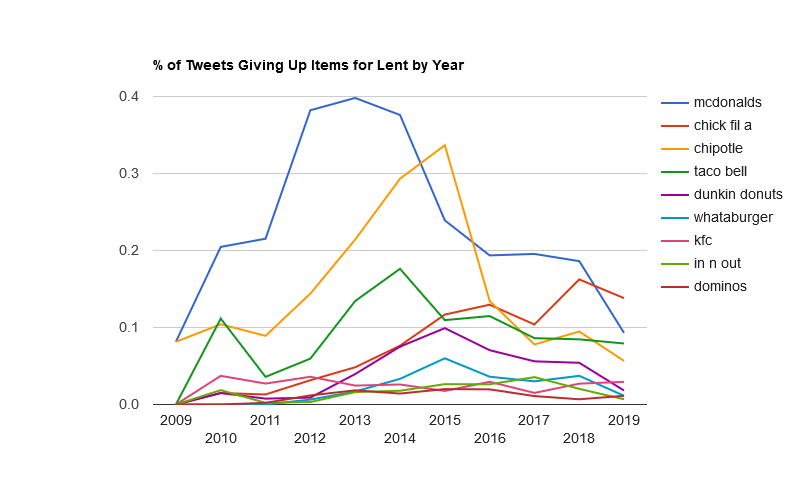
Other Updates from Last Year
Hot Cheetos finally declined. Smoking and Juuling both rose. Tide Pods look to be a one-year phenomenon, along with Fortnite. Snapchat dropped off a cliff.
Top 100 Things Twitterers Gave Up for Lent in 2019
1. | Social networking | 1,529 | 0 |
2. | Alcohol | 1,498 | +1 |
3. | 1,409 | -1 | |
4. | Chocolate | 818 | 0 |
5. | Lent | 770 | +6 |
6. | Meat | 684 | 0 |
7. | Swearing | 606 | -2 |
8. | Coffee | 563 | +1 |
9. | Soda | 561 | -1 |
10. | Sex | 511 | +3 |
11. | Fast food | 473 | -1 |
12. | Sweets | 460 | -5 |
13. | School | 414 | +2 |
14. | Men | 374 | +6 |
15. | Work | 367 | +11 |
16. | College | 346 | +9 |
17. | Religion | 346 | +15 |
18. | Bread | 336 | -4 |
19. | You | 327 | 0 |
20. | Plastic | 312 | 0 |
21. | Sugar | 294 | 0 |
22. | Catholicism | 290 | +15 |
23. | Giving up things | 289 | +10 |
24. | Beer | 274 | -6 |
25. | Chips | 269 | -9 |
26. | Life | 258 | +3 |
27. | 227 | -15 | |
28. | Marijuana | 224 | +3 |
29. | Brexit | 212 | +47 |
30. | Boys | 204 | -8 |
31. | 195 | -4 | |
32. | Virginity | 187 | +28 |
33. | Smoking | 175 | +7 |
34. | Candy | 161 | -11 |
35. | Starbucks | 144 | -1 |
36. | Junk food | 138 | -3 |
37. | Hope | 128 | +22 |
38. | Homework | 128 | +8 |
39. | Rice | 127 | +8 |
40. | Breathing | 125 | +32 |
41. | Cheese | 122 | -5 |
42. | Donald Trump | 122 | +18 |
43. | Red meat | 121 | -8 |
44. | Lying | 118 | 0 |
45. | Food | 113 | +24 |
46. | Wine | 111 | -5 |
47. | Carbs | 111 | -6 |
48. | Winter | 110 | +48 |
49. | Fried food | 109 | +1 |
50. | Masturbation | 109 | +6 |
51. | Gossip | 108 | +41 |
52. | Depression | 105 | +26 |
53. | Anxiety | 103 | +30 |
54. | Ice cream | 103 | -4 |
55. | My job | 103 | +26 |
56. | Him | 98 | +10 |
57. | Cookies | 98 | -5 |
58. | Pizza | 96 | -20 |
59. | People | 94 | -5 |
60. | Dairy | 94 | -15 |
61. | Booze | 92 | -13 |
62. | Procrastination | 91 | -3 |
63. | Single use plastic | 84 | -10 |
64. | Eating out | 84 | 0 |
65. | Liquor | 81 | -7 |
66. | Juuling | 80 | -1 |
67. | Boba | 79 | +4 |
68. | Christianity | 76 | +15 |
69. | Takeout | 75 | -10 |
70. | Caffeine | 75 | -15 |
71. | Shopping | 74 | -17 |
72. | Negativity | 73 | -46 |
73. | My will to live | 69 | -1 |
74. | Sobriety | 68 | -16 |
75. | Online shopping | 66 | -1 |
76. | Bills | 64 | +19 |
77. | French fries | 64 | -16 |
78. | Lint | 63 | +6 |
79. | Chick Fil A | 61 | -16 |
80. | Complaining | 61 | -29 |
81. | Sleep | 61 | -11 |
82. | Desserts | 60 | -15 |
83. | Church | 60 | -5 |
84. | Coke | 59 | -16 |
85. | Pussy | 59 | -6 |
86. | Hot Cheetos | 56 | -25 |
87. | Netflix | 55 | -25 |
88. | God | 54 | -3 |
89. | Porn | 53 | -24 |
90. | Snapchat | 50 | -73 |
91. | Stress | 50 | -3 |
92. | Oxygen | 50 | +4 |
93. | Spending | 50 | -3 |
94. | Pancakes | 46 | -21 |
95. | Crying | 46 | -1 |
96. | Diet coke | 46 | -19 |
97. | Juice | 45 | -15 |
98. | Chicken | 44 | -19 |
99. | Cheating | 43 | -19 |
100. | F***boys | 43 | -43 |
Top Categories
This year, the top celebrity was BTS, a Korean boy band / all-consuming lifestyle.
1. | food | 8,004 |
2. | technology | 3,688 |
3. | habits | 2,963 |
4. | smoking/drugs/alcohol | 2,820 |
5. | irony | 2,097 |
6. | relationship | 1,800 |
7. | school/work | 1,490 |
8. | sex | 1,164 |
9. | religion | 1,016 |
10. | politics | 440 |
11. | generic | 427 |
12. | money | 353 |
13. | health/hygiene | 348 |
14. | entertainment | 224 |
15. | shopping | 182 |
16. | weather | 171 |
17. | sports | 165 |
18. | possessions | 54 |
19. | celebrity | 24 |
20. | clothes | 16 |
Media Coverage
The Lent Tracker received some media attention this year:
- Christianity Today
- Minneapolis Star Tribune (this reporter actually interviewed me)
- Relevant Magazine
- Christian Post
- The Mercury News
- ABC6 Philadelphia, ABC7 Chicago, and Fox 8 New Orleans (slideshows)